5 Keys to Establishing and Maintaining Optimal Target Inventory Levels
At a high level, a supply chain’s structure defines critical factors affecting optimal target inventory levels – factors such as replenishment lead time and variation, customer demand and variation, and replenishment frequency. Supply-chain structure is fundamental to optimal overall financial performance. Still, to achieve target service levels reliably with minimal inventory and expediting, we must establish and maintain optimal target inventory levels for each item in each stocking location.
So we ask: Can you establish and maintain the optimal inventory position for each item in each location?
It’s a heavy question, containing these 5 key sub-topics:
- Optimal inventory – definition
- Target inventory levels
- Importance of optimal target inventory levels by Item-AND-Location
- Establish optimal target inventory levels
- Maintain optimal target inventory levels
We’ll next discuss each one. We’ll also introduce real-world challenging concepts that are vital to optimal inventory levels – and how to address them correctly and reliably.
1. Optimal:
Stating the obvious: Optimal inventory strikes the perfect balance between our customers’ fulfillment expectations and our overall financial performance. This balance must reflect monetary value, intangible perceptions, market share and penetration, pricing premiums, hard costs, soft costs – and the list goes on. For your company, even for your individual product families, the relevant factors and their relative weights may well combine in a way different than any other business. There is simply no one-size-fits-all formula or calculation for optimally balancing inventory levels and customer fulfillment.
However, after considering your various applicable factors and their weights, you can represent your ideal balance in just one number: Target service level (TSL) – a fill rate quantifying your unique, optimal balance of factors affecting your customers and your financial performance.
You may have different TSLs for certain products, product families and inventoried components making up those products. You may also have different TSLs for inventory-stocking locations – warehouses, in-process inventory, DCs, outlets, etc.
Given everything above, our definition of optimal inventory is the lowest feasible target inventory level that consistently meets your TSL.
––––––––––––––––––––––––––––––––––––––––––––––––––––––––––––––––––––––––
Challenging concept: You and your customers easily understand TSL. Yet in your real world, customers are sensitive not only to TSL, but also to your consistency in achieving it.
Consider a service-level agreement (SLA) example: You and customer ABC have an SLA requiring a 99% fill-rate TSL, measured quarterly – and it must be achieved for 10 quarters out of 12.
This concept is called confidence: Achieving TSL reliably – in this case, 83% confidence (10 out of 12 quarters) of achieving 99% TSL. Maybe yours is not a formal SLA environment. Nonetheless, your customers implicitly expect both a certain TSL and confidence of its consistency.
So confidence is important in the real world. The challenge lies in how to factor confidence, correctly and objectively, into optimal target inventory levels. The solution involves a probabilistic analysis, not a deterministic algorithm.
––––––––––––––––––––––––––––––––––––––––––––––––––––––––––––––––––––––––
2. Target Inventory Levels:
For an item in a location, we define target inventory level as the average quantity on hand, or AQOH. AQOH is critical for optimally balancing customer fulfillment and financial performance. We’ll review a time-tested heuristic to estimate AQOH in a minute, but first we need to cover other critical target inventory settings since AQOH alone is not what triggers replenishment.
Instead, an item’s replenishment parameters drive optimal target inventory levels (optimal AQOH). Each item-and-location’s replenishment method dictates its specific replenishment parameters. AQOH is simply the result. Any replenishment method or system contains assumptions and often calculations based on input factors, including lead time, reorder quantity (ROQ) and expected future demand behavior.
Whatever approach you use to establish and maintain optimal target inventory levels, you must ensure it can support multiple replenishment methods to provide analytical flexibility. To drive the right target inventory levels – optimally established and maintained within your supply chain – a replenishment method that consistently achieves TSL requires these replenishment parameter(s):
Replenishment Method Parameter 1 Parameter 2
MRP . . . . . . . . . . . . . . . . . . . . . Safety Stock . . . . . . . . (none)
ROP . . . . . . . . . . . . . . . . . . . . . Reorder Point . . . . . . . (none)
Min-Max . . . . . . . . . . . . . . . . . Min . . . . . . . . . . . . . . . Max
Kanban, “Two-Bin” . . . . . . . . Quantity per Bin . . . . . (2 bins, obviously)
Kanban, “Multi-Card” . . . . . . Quantity per Card . . . Number of Cards
PAR Level . . . . . . . . . . . . . . . . PAR Level . . . . . . . . . . (none)
Here’s a practical heuristic for approximating optimal target inventory levels:
AQOH = 50% of Reorder Quantity + Buffer Inventory
(Note: Buffer Inventory is incremental stock for achieving TSL with expected confidence. It’s interchangeable with “safety stock.” For MRP users, “safety stock” may carry negative implications involving MRP replenishment suggestions, bullwhip effect, etc.)
The heuristic’s 50% of Reorder Quantity is easy. Reorder quantity, ROQ, typically results from an MOQ, EOQ, or fixed reorder-review frequency. For a specific location, inventory item 123’s supplier may say MOQ = 1000. Or you determine that EOQ = 1200. Or you review 123’s replenishments every two weeks, and 123’s average demand during this interval = 1350.
The heuristic’s Buffer Inventory is difficult to quantify, being influenced even by replenishment method:
- MRP – safety stock (or perhaps safety time) is the buffer
- Two-bin kanban – a portion of buffer in each bin
- Multi-card kanban – additional cards are the buffer
- ROP, min-max, PAR – the first (or only) inventory-policy parameter includes buffer
––––––––––––––––––––––––––––––––––––––––––––––––––––––––––––––––––––––––
Challenging concept: Any item-and-location’s replenishment method affects its buffer. When we analyze each replenishment method’s optimal buffer for a given item-and-location, each method’s buffer may be different.
Optimal buffer is vital to optimal target inventory levels, and optimal target inventory levels come from effective replenishment parameters.
A deterministic formula cannot accommodate the differences in replenishment methods. Consider, for example, a “what-if” scenario for an inventory item that we may want to manage by either “two-bin” kanban or min-max. Experimenting with the AQOH heuristic shows us that “two-bin” kanban, though very simple to execute, typically drives a higher AQOH. It simply doesn’t have the inventory-velocity efficiency of min-max.
Part of this AQOH difference results from the ROQ portion of the AQOH heuristic. But two-bin-kanban’s buffer inventory is also different than that required for min-max. Deterministic safety-stock algorithms assume a replenishment method of classic ROP, and do not address replenishment-method differences.
Even a probabilistic analysis must be structured properly to reflect the executional differences between methods. Really, such an analysis must be a simulation. Our supply-chain experience tells us that significant replenishment-signaling and -execution differences exist between replenishment methods. For example, kanban, min-max and even PAR level are all variations on the fundamental ROP replenishment method – but each is different, often providing a different balance of executional simplicity and inventory-velocity performance.
––––––––––––––––––––––––––––––––––––––––––––––––––––––––––––––––––––––––
3. Importance of Optimal Target Inventory Levels by Item-and-Location:
Actual service-level performance happens not just for each item, but also at each location. Tell a customer that he’ll need to go get his order at a different location than expected, or ask him to wait for his order to come to the current location, and he will view this as a service-level failure.
To minimize or hide the fulfillment delay, you could expedite from another location. But this reduces your financial performance. In this strictest sense, supply-chain structure has no relation to TSL, which succeeds or fails for an item in a location.
Supply-chain structure defines certain factors that determine optimal target inventory levels – factors such as replenishment lead time and variation, customer demand and variation, and replenishment frequency. Supply-chain structure is key to optimizing overall financial performance, and many more factors go into an optimal supply-chain structure than just optimal inventory. Still, given that the factors determining optimal target inventory levels often change by location for a given item, we then must establish and maintain optimal target inventory levels for each item in each stocking location.
––––––––––––––––––––––––––––––––––––––––––––––––––––––––––––––––––––––––
Challenging concept: Your item-and-location combinations may present a daunting analytical effort:
- How to identify, gather and analyze the required data – another in-house ad hoc spreadsheet?
- What optimization algorithm or analysis to use?
- Can a simple heuristic or rule of thumb get “close enough”?
- How often to update the analysis?
- How to know when the prior analysis is out-of-date?
- What skill sets are required?
Optimizing each item-and-location’s target inventory level requires a higher-level analysis and more data. Spreadsheets, of course, can handle intense analysis and extensive input data, but their computational overhead makes them slow. On the other hand, available heuristics and rules of thumb are too simplistic and generalized truly to optimize a supply chain of any complexity.
––––––––––––––––––––––––––––––––––––––––––––––––––––––––––––––––––––––––
4. Establish Optimal Target Inventory Levels:
Bottom line: we don’t believe that any static calculation or formula can achieve optimal target inventory levels. Such algorithms cannot accommodate the many relevant input factors and their variability across items, or for the same item across locations, making their effectiveness inconsistent at best. In addition, any calculation by definition uses the same measure of variability for all items – regardless of the real patterns of actual variability that can be determined by more closely examining each item’s past behavior.
We advocate modeling supply-chain behavior by simulation – for any item in any location – using the input factors we discussed previously. A correctly-structured supply-chain model shows replenishment receipts, demand (or usage) fulfillments and resulting on-hand inventory levels. By using such a model to simulate any item-and-location’s supply-chain behavior, you may then objectively conclude the optimal target inventory level that would have achieved your TSL during the model’s service-level-cycle time period. Next, you should run many comparative iterations of this simulation model – with varied inputs – to test the validity of the optimal inventory targets identified by the model.
A note on variation: We know intuitively that random demand-and-supply variations pose the greatest threats to TSL. Just what is random variation?
Forecasting involves several variation types: Trend, seasonality and special causes – promotions, product introductions and phase-outs, etc. Our forecast includes them because:
- We can reasonably estimate variation magnitude and behavior, AND
- We can reasonably estimate variation timing
In contrast, random variation has unpredictable timing. We cannot forecast “when,” so we must cover the random variation with “extra” on-hand inventory. (Expediting is an unlikely alternative – possible only if we can expedite without unfavorable financial consequences.)
Though we cannot predict random variation’s timing, we can predict its future behavior and magnitude – so long as an item-and-location’s demand-and-supply process is stable. “Stable” does not mean “minimal variation.” Indeed, a stable process may have dramatic variation. Stable simply means past random-variation behavior and magnitude will continue in the future.
To establish each item-and-location’s optimal inventory position, we must first define – objectively, mathematically and statistically – the behavior and magnitude of an item-and-location’s random demand-and-supply variations. Our best source is historical actual data, such as sales-order detail or inventory-consumption transactions. When we don’t have reliable hard data, we use our best source of estimates, such as our experienced buyers, planners and schedulers.
Modeling supply chain behavior by simulation reveals interesting relationships between required inventory buffers and input factors — relationships that aren’t necessarily intuitive and that aren’t correctly handled by textbook inventory formulas, such as:
- MOQ, EOQ, fixed replenishment frequency: Large MOQs aren’t ideal, but their resulting large quantities on hand provide de facto buffer inventory. Likewise, small MOQs mean frequent replenishments that provide de facto buffer – a steady queue of inbound receipts about to arrive.
- Past-due backlog vs. unfulfilled-order cancellation: When you don’t fulfill a customer’s order on time, he may cancel it. Or it may become past-due backlog until fulfilled. Past-due demand is disruptive, causing other orders to become past-due. As an item-and-location’s probability of past-due backlog goes up, it requires more buffer inventory to achieve TSL consistently.
- Service-level cycle: Your supply-chain KPIs include an actual fill rate (e.g., on-time delivery %) during a cycle (e.g., a quarter). It’s harder to achieve this KPI consistently if it’s measured for a month instead of a quarter. Shorter cycle = more volatile results. You need more buffer to achieve your TSL consistently every month than every quarter. Service-level cycle is a critical factor in optimizing each item-and-location’s inventory position.
––––––––––––––––––––––––––––––––––––––––––––––––––––––––––––––––––––––––
Challenging concept: Certainly, you can develop queries to extract and compile item-and-location information: TSL, TSL confidence, historical actual demand, actual or estimated replenishment lead times and variation, MOQs, probability of past-due backlog and service-level cycle. Now – what to do with all of this data?
Clearly, creating a fast, robust and correct probabilistic simulation of inventory behavior at the item-and-location level is no small task. However, achieving reliable and optimal target inventory levels requires exactly this type of simulation.
––––––––––––––––––––––––––––––––––––––––––––––––––––––––––––––––––––––––
5. Maintain Optimal Target Inventory Levels:
You can optimize item-and-location inventory position as a one-time project. But you won’t reap long-term, ongoing optimization. Your business – and your supply chain – are dynamic.
Challenging concept: Today’s optimal inventory may also apply to tomorrow, next week or even next month. But probably not next quarter or year. So how often to update each item-and-location? A fixed frequency – once per quarter? Do KPIs exist indicating that a given item, product family, location, etc. needs an update?
How often? Two answers:
- You know your supply chain. This helps you determine a basic inventory-position update interval. It’s typically a quarter. But your knowledge and experience may indicate more or less frequently.
- A correct, comprehensive inventory-and-fulfillment simulation provides upper and lower expected bounds, control limits of a sort, for a spectrum of demand and supply KPIs. If an item-and-location exceeds its bounds, its dynamic supply-chain parameters need to be updated and re-analyzed. Those bounds objectively suggest that something in the item’s demand-and-supply process has changed – not randomly, but significantly.
––––––––––––––––––––––––––––––––––––––––––––––––––––––––––––––––––––––––
Clearly, a solution which provides these 5 keys to establishing and maintaining optimal inventory position, for each item in each location, is not easily constructed. However, the supply-chain world is progressively adopting and applying the power of predictive analytics. With today’s analytical capabilities and server technologies, supply chain modeling and iterative simulation that would take 2.5 hours in a spreadsheet can now be done in less than 5 seconds. That said, 5 seconds multiplied by tens of thousands of items is still a chunk of time. Fortunately, such a solution doesn’t require daily updates – it need not be live and real-time like a perpetual-inventory system.
Such a probabilistic simulation – mathematically and statistically correct, comprehensively representing real-world inventory / demand / supply behavior, fast and robust – is available commercially. In preparation for using such a viable supply-chain-optimization solution, and as an important exercise in discovery, we recommend that you construct your own ad hoc item-and-location supply-chain simulation. It will provide you with valuable insight, clarifying critical factors and behaviors of your unique supply chain that may currently be obscured by departmental bounds and existing as undocumented intuition.
By David McPhetrige
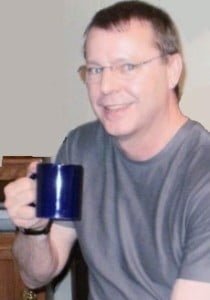
David has over 30 years of experience in lean and flow, MRP, information technology, cost accounting and finance across a broad range of industries. Since 1994, he has implemented lean and flow worldwide in scores of facilities and industries, including regulated environments.
David holds two patents involving optimal inventory, and is the inventor of the analytical technology and methodology behind Right Sized Inventory LLC’s comprehensive inventory-optimization solution.
David has implemented multiple MRP/ERP systems, and has led, driven and developed many post-implementation MRP/ERP enhancements. He has managed cost accounting in both work-order and repetitive-manufacturing environments, and has been Director of Finance in a division of Emerson Electric.
Connect with David on LinkedIn | View the Right Sized Inventory page on LinkedIn